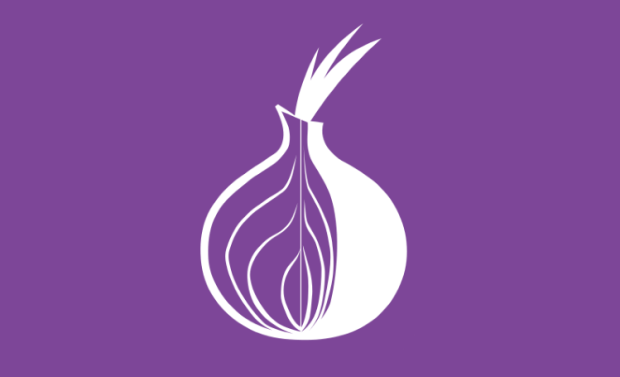
A new machine learning algorithm is able to detect the use of apps like YouTube, Instagram, among others, in Tor browser
A group of network security and ethical hacking specialists claims to have developed an algorithm capable of detecting the activity patterns in Android operating system apps within the Tor network traffic with 97% accuracy.
This algorithm is not an anti-anonymity script, because it cannot reveal the real IP address of a user, nor other details about their identity. Still, this algorithm is able to reveal if a Tor user is using an Android application.
The work of this team of experts in network security is based on previous developments able to analyze the flows of TCP packets of traffic in Tor and differentiate between eight different types of traffic: browsing, chatting, email, streaming audio, video, File transfer, VoIP and P2P.
This time the specialists applied a similar concept of analysis of TCP packets flowing through a Tor connection to detect specific patterns associated with the activity of certain Android applications.
Subsequently, they developed a machine learning algorithm trained with Tor traffic patterns from ten different apps: Android Tor Browser, Facebook, Instagram, Twitter, YouTube, Spotify, Skype, Twitch, Replaio Radio and DailyMotion.
Once the algorithm training was completed, the specialists used it in Tor traffic to detect whether an individual were using any of these apps. The results were overwhelming, the algorithm showed 97.3% accuracy.
However, the algorithm is not as efficient as it seems. According to network security specialists from the International Institute of Cyber Security, it can only be used when there is no background traffic, in other words, when the user is using one and only one application on a smart device.
This means that when there are two or more apps communicating at the same time in the background, the algorithm starts confusing TCP traffic patterns, so its effectiveness level decreases.
In addition, the algorithm also has precision flaws. Some streaming apps (like YouTube or Spotify) produce very similar traffic patterns, so the algorithm tends to confuse them.
Finally, as future experiments consider the analysis of more applications, similar problems will continue to appear, thus the effectiveness of the algorithm will be considerably reduced.
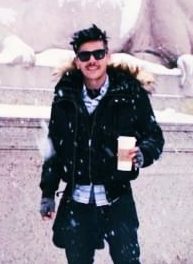
He is a well-known expert in mobile security and malware analysis. He studied Computer Science at NYU and started working as a cyber security analyst in 2003. He is actively working as an anti-malware expert. He also worked for security companies like Kaspersky Lab. His everyday job includes researching about new malware and cyber security incidents. Also he has deep level of knowledge in mobile security and mobile vulnerabilities.