A team of cybersecurity specialists published their findings on a new approach based on the analysis of the irradiation of electromagnetic fields in Internet of Things (IoT) devices, which would allow the identification of complex malware variants in these systems.
For years, IoT devices have become a favorite target of threat actors, as features such as the lack of advanced security mechanisms and the use of common firmware make them highly vulnerable to various variants of cyberattacks.
The technique described by this team would allow malware analysts to determine exactly what type of malicious software is infecting an IoT device, regardless of whether the code is obfuscated to evade detection and using only side-channel information.
Experts say that the electromagnetic field irradiation that is measured from the device is practically undetectable for malware, so their evasion techniques do not apply in these readings: “Since malware has no control over external events at the hardware level, a monitoring and security system based on hardware components cannot be disabled, not even by the most advanced malware samples.”
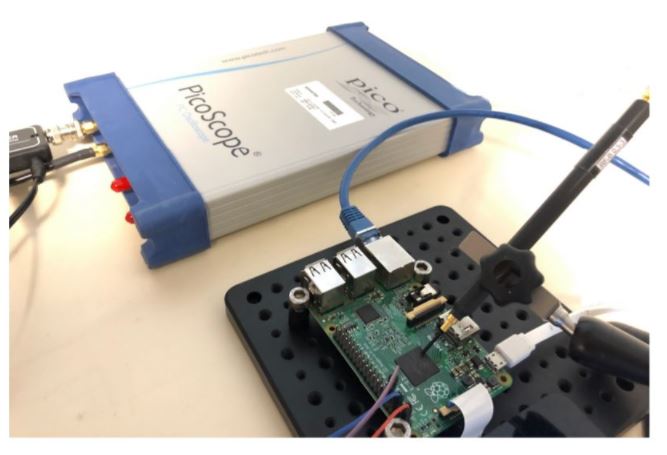
The best part of this finding is that applying such a security approach requires no modifications to IoT devices: “We tested by analyzing a Raspberry Pi using harmless code and malicious payloads. We use an oscilloscope with 1GHz bandwidth (Picoscope 6407) connected to an H-Field Probe (Langer RF-R 0.3-3), where the electromagnetic signal is amplified using a Langer PA-303 + 30dB. To capture prolonged malware execution, the signals were sampled at a sample rate of 2MHz,” the experts add.
The data collected can be noisy, so the researchers also implemented a processing stage to isolate the signals relevant to the analysis. This information was used to train neural network models and machine learning algorithms, responsible for classifying malware types, binaries, and obfuscation methods.
In total, the researchers collected about 3,000 traces corresponding to 30 malware binaries and 10,000 traces of non-malicious activity. This approach makes it possible to predict the presence of at least three types of generic malware on IoT devices with 99% accuracy, the experts concluded.
To learn more about information security risks, malware variants, vulnerabilities and information technologies, feel free to access the International Institute of Cyber Security (IICS) websites.
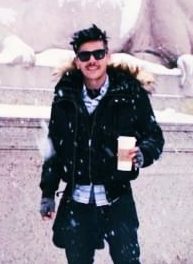
He is a well-known expert in mobile security and malware analysis. He studied Computer Science at NYU and started working as a cyber security analyst in 2003. He is actively working as an anti-malware expert. He also worked for security companies like Kaspersky Lab. His everyday job includes researching about new malware and cyber security incidents. Also he has deep level of knowledge in mobile security and mobile vulnerabilities.